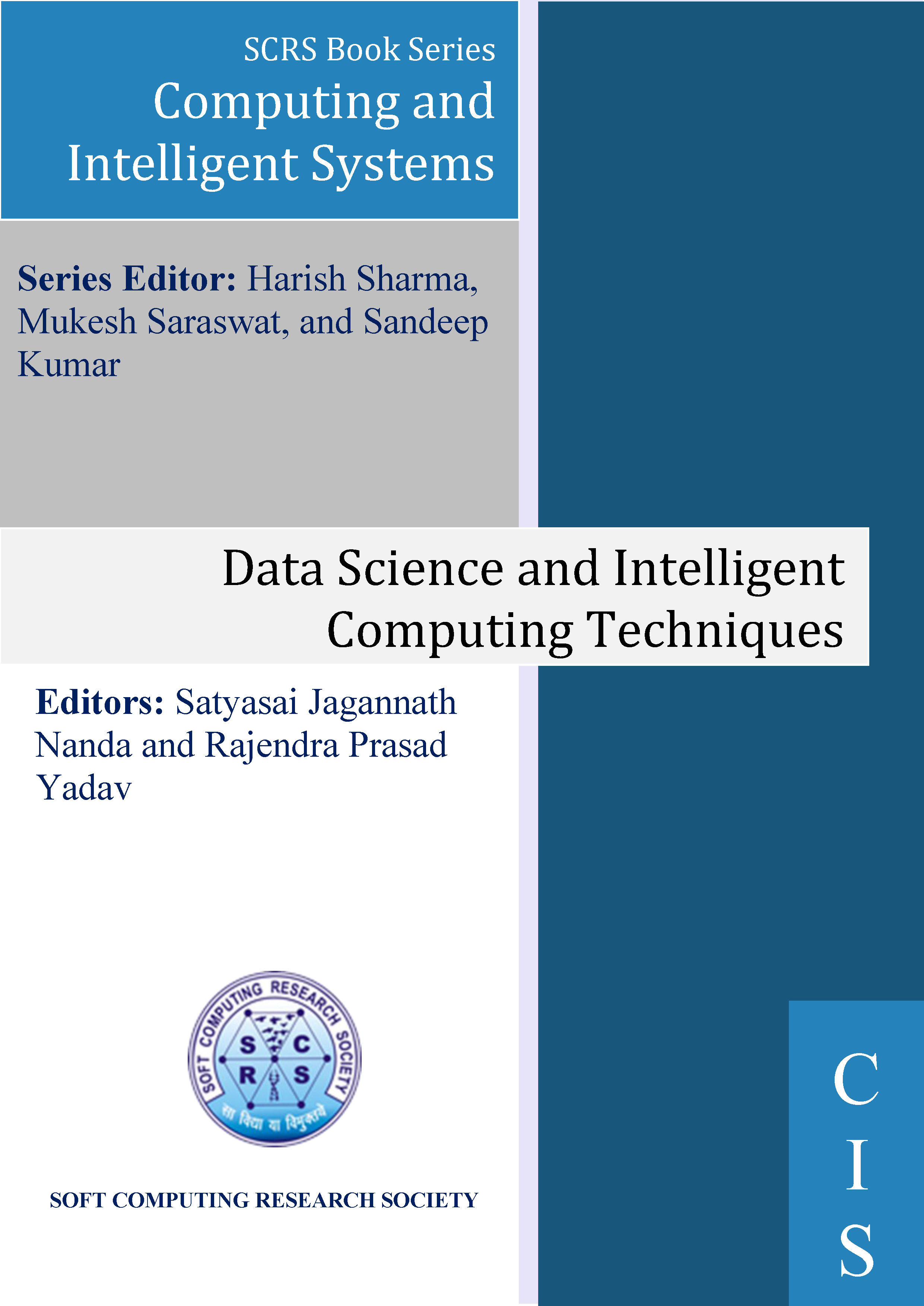
A Novel Approach for Crop Yield Prediction Model Validation
Authors: Moumita Goswami, Sanghita Bhattacharjee and Suvamoy Changder
Publishing Date: 12-01-2023
ISBN: 978-81-955020-2-8
Abstract
Agriculture is the backbone of the Indian economy, which is mainly dependent on nature. Nowadays, due to the random climatic changes, farmers are struggling to get a good amount of yield from the crops. Smart Agriculture is one of the crop management concepts that allows farmers to control the farm in all aspects. Crop prediction is a challenging task in the area of agriculture to increase crop productivity. Machine learning plays a vital role in crop yield prediction, including supportive decisions on what crops to produce. The main objective of this paper is to provide a double filter mechanism to focus on the validation of the model, thus predicting the suitable crops to be farmed on. Several supervised machine learning classifiers have been applied in our work for predicting an appropriate crop based on different soil and weather parameters. When the accuracy of the classifiers is almost the same, it becomes difficult to identify a proper classifier for predicting suitable crops. In our work, we have focused on the validation of the classifier model which further helps us to find the appropriate classifier to support crop yield prediction. The clustering algorithm is used for testing and validating the correctness of the proper classifier. The experimental results show that every model is not applicable in all the cases of crop prediction. Hence there is a gap between model prediction and actual implementation. This paper bridges the gap between the selection procedure.
Keywords
Agriculture, Classification algorithms, Accuracy, Validation, Clustering algorithm, Clusters
Cite as
Moumita Goswami, Sanghita Bhattacharjee and Suvamoy Changder, "A Novel Approach for Crop Yield Prediction Model Validation", In: Satyasai Jagannath Nanda and Rajendra Prasad Yadav (eds), Data Science and Intelligent Computing Techniques, SCRS, India, 2023, pp. 103-116. https://doi.org/10.56155/978-81-955020-2-8-10