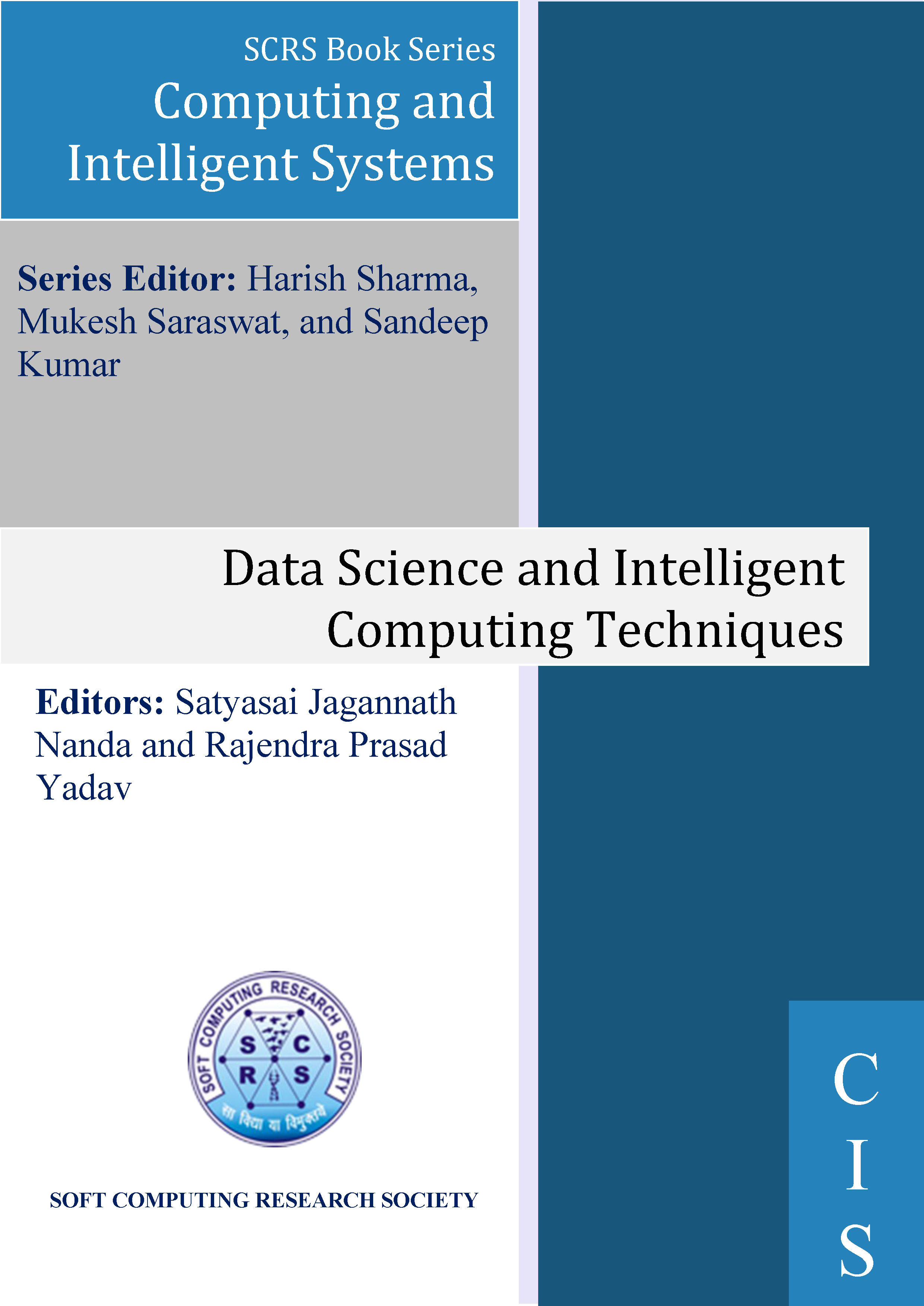
Pragmatic Analysis of Vein Detection and Processing Models from an Empirical Perspective
Authors: Manisha A. Gawande and Suchita W Varade
Publishing Date: 17-10-2023
ISBN: 978-81-955020-2-8
Abstract
Vein detection is a difficult process that can be carried out using context-dependent intrusive & non-intrusive techniques. The models based on image processing, pressure sensing, depth detection, etc. are just a few examples of these techniques. Finding the best vein detection model for a given deployment is challenging, especially when evaluated in terms of their computing complexity, deployment costs, accuracy, precision, and other context-specific criteria. The ambiguity of model selection for various use-cases is further increased by these variances in model performance. Due to this ambiguity, researchers and clinical system designers are required to test & validate different detection models, before using them for their application-specific deployments. To reduce this ambiguity, in identification of an optimum models for This essay contrasts various vein identification techniques in terms of various processing & deployment properties based on distinct use cases. It was discovered that Machine Learning Models (MLMs), including Nave Bayes (NB), Recurrent Neural Networks (RNNs), and Convolutional Neural Networks (CNNs), outperformed their competitors. These models use feature augmentation with effective classification in order to optimize Vein detection performance under different clinical scenarios. In order to facilitate decision-making, this paper compares various models' detection accuracy, precision, recall, computational delay, deployment cost, and scalability metrics. Additionally, this paper suggests evaluating a Vein Model Rank Score (VMRS), which integrates various evaluation metrics to identify models with superior all-around performance. Based on VRMS, researchers will be able to identify methods that have better accuracy, lower delay, and higher viability of deployment under clinical use cases.
Keywords
Vein Detection, Machine Learning, Intrusive, Non-Intrusive, Accuracy, Precision, Complexity, Vein Model Rank Score.
Cite as
Manisha A. Gawande and Suchita W Varade, "Pragmatic Analysis of Vein Detection and Processing Models from an Empirical Perspective", In: Satyasai Jagannath Nanda and Rajendra Prasad Yadav (eds), Data Science and Intelligent Computing Techniques, SCRS, India, 2023, pp. 381-391. https://doi.org/10.56155/978-81-955020-2-8-34