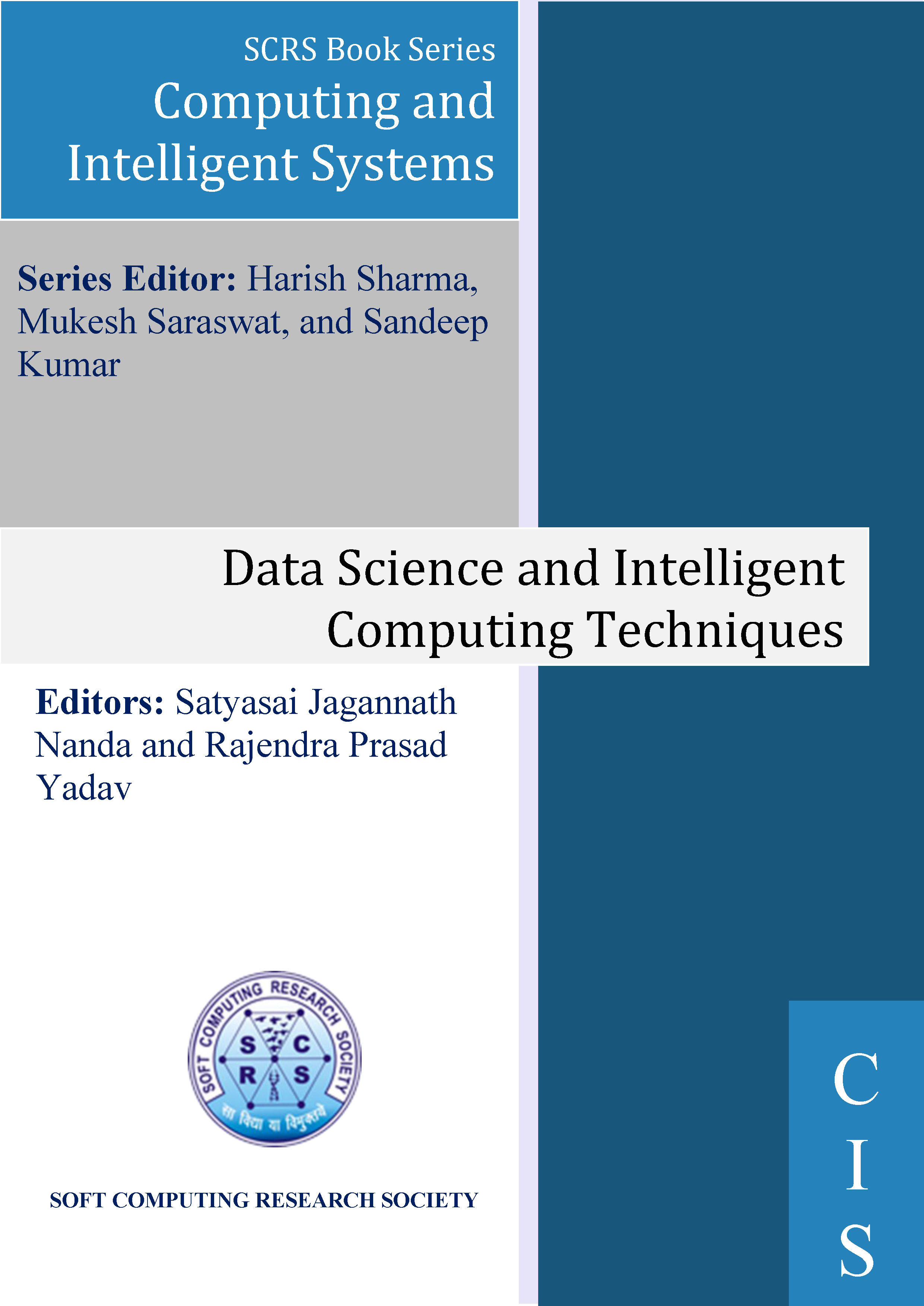
Streamlining Tax Calculations: An Automated Approach for Individual Taxpayer
Authors: Sahil Saurabh Jain, Swati Sharma and Anuj Kumar Bharti
Publishing Date: 17-10-2023
ISBN: 978-81-955020-2-8
Abstract
In this paper, we provide an innovative tax computation method that intends to automate and simplify the calculation of taxes for Indian citizens. The method that we suggest in this work uses machine learning (ML), natural language processing (NLP), optical character recognition (OCR), and OCR technologies to accurately interpret financial data from a user's bank statement. The method we provide here leverages and employs data extraction, cleaning, sorting, and categorization using NLP techniques, as well as tax computation based on the current Indian tax framework. Our approach tackles both the shortcomings of current automated tax computation systems as well as the difficulties Indian taxpayers encounter when navigating the complicated tax structure of their nation. With the help of automation of the tax calculation process, we aim to reduce the likelihood of errors and minimize the time and effort required by taxpayers while preventing fraudulent activities. Although our approach has shown promising results, there are limitations and areas for further development, such as improving OCR accuracy, adding support for different languages, managing complex tax scenarios (for people owning business), enhancing scalability, and addressing privacy and security concerns. With further research and development, our proposed tax computation method has the potential to streamline and enhance the tax filing process in India, making it more efficient, accurate, and user-friendly.
Keywords
OCR, Tax calculation, Natural Language Processing, Machine Learning.
Cite as
Sahil Saurabh Jain, Swati Sharma and Anuj Kumar Bharti, "Streamlining Tax Calculations: An Automated Approach for Individual Taxpayer", In: Satyasai Jagannath Nanda and Rajendra Prasad Yadav (eds), Data Science and Intelligent Computing Techniques, SCRS, India, 2023, pp. 401-410. https://doi.org/10.56155/978-81-955020-2-8-36