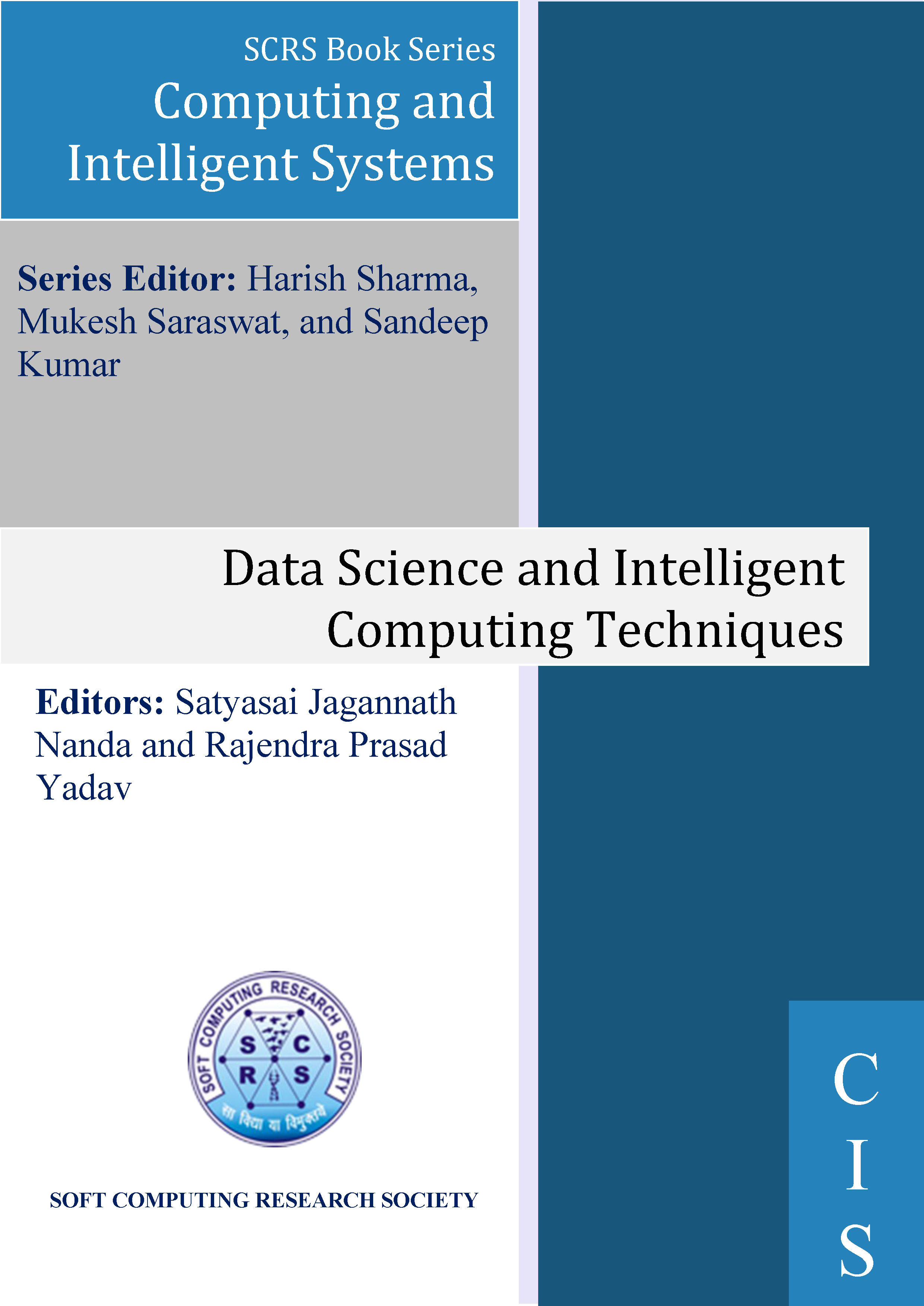
Early Detection of Cardiovascular Diseasesin ECG Images Using Convolutional Neural Networks
Authors: Jhansi Bheemapalli and V. Rama
Publishing Date: 05-11-2023
ISBN: 978-81-955020-2-8
Abstract
Cardiovascular diseases (CVDs) account for nearly a third of all deaths globally yearly. So, early detection and type can greatly reduce the death charge by assisting in providing early and proper medication. Electrocardiogram (ECG) is a non-invasive tool, used to file the electrical hobby of the heart at normal durations. In this paper, a Convolutional neural network (CNN) is used to expect four training of sufferers: peculiar heartbeat, myocardial infarction, records of myocardial infarction, and everyday heartbeat using the general public ECG photos dataset of cardiac sufferers. The statistics used for schooling are not multiplied to large portions by any information augmentation strategies to maintain the first-class training facts. The CNN technique is investigated by way of schooling with different optimizers like Stochastic Gradient Descent with Momentum (SGDM), Root implies square Propagation (RMS-Prop), and Adaptive moment Estimation (Adam). Then, CNN is investigated through training with various initial learning rates of 0.001, 0.01, and 0.1. In keeping with the experimental consequences, the CNN with SGDM solver with aninitial learning rate of 0.1 gave an excellent validation accuracy of 95.29% in comparison to existing work with Adam as solver with an accuracy of 92.18%.
Keywords
Cardiovascular Diseases, Electrocardiogram, Convolutional Neural Network, Optimizers, Initial learning rates.
Cite as
Jhansi Bheemapalli and V. Rama, "Early Detection of Cardiovascular Diseasesin ECG Images Using Convolutional Neural Networks", In: Satyasai Jagannath Nanda and Rajendra Prasad Yadav (eds), Data Science and Intelligent Computing Techniques, SCRS, India, 2023, pp. 623-632. https://doi.org/10.56155/978-81-955020-2-8-56