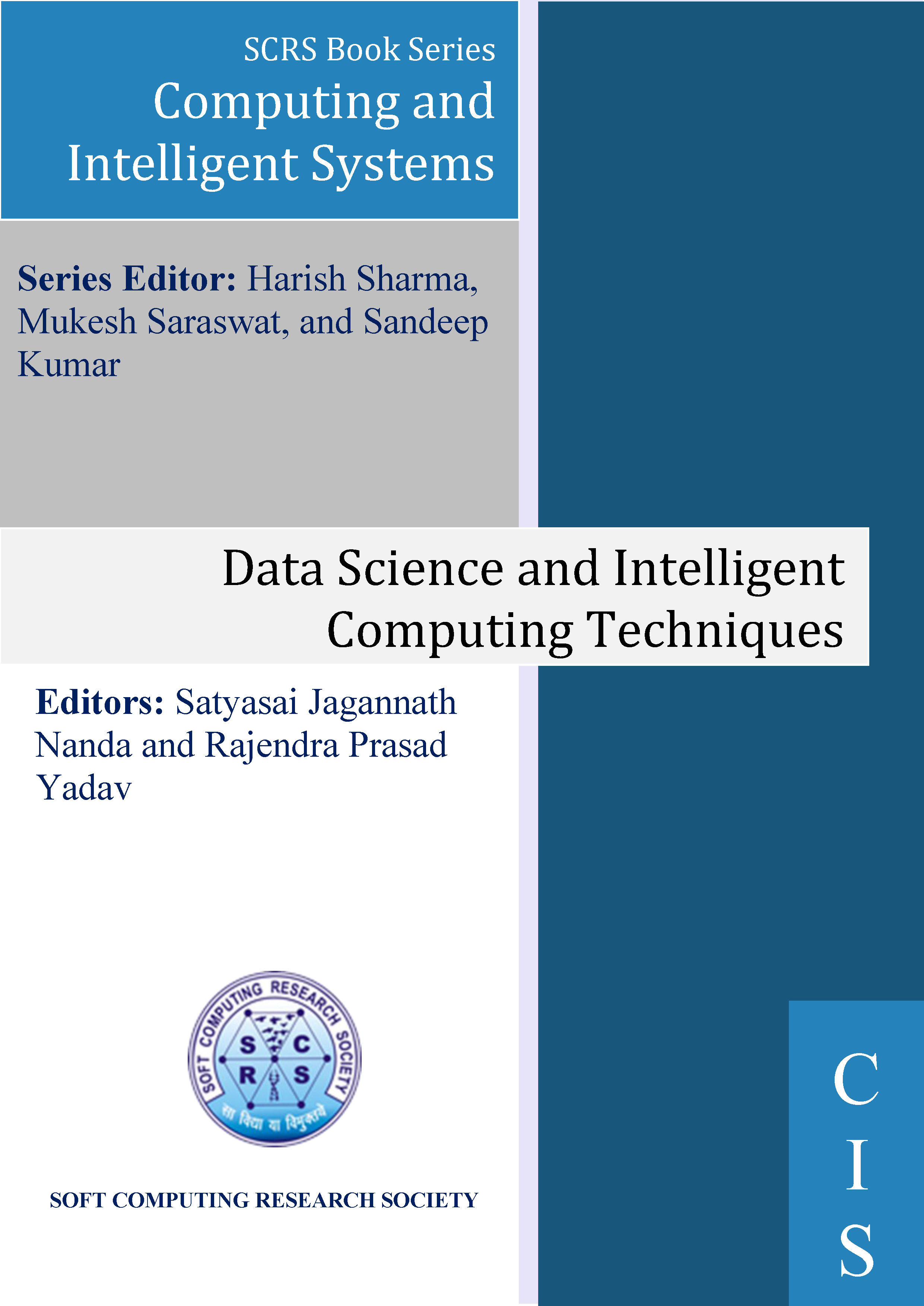
Enhancing Precision Agriculture Through Novel Soil Nutrient Prescription Recommender System Using Quantile Regression Forests
Authors: Sareena Rose, S Nickolas and S Sangeetha
Publishing Date: 09-12-2023
ISBN: 978-81-955020-2-8
Abstract
The conception of a soil nutrient-specific prescription recommender system will streamline the understanding of the cause-and-effect relationships inherent to di- verse nutrients and help in informed decision-making about farming methodolo- gies. Prescriptive analytics proposes optimal solutions Using Quantile Regression Forests and provides recommendations that enable soil scientists to understand the sufficiency/deficiency of soil nutrients at customised locations. Unlike traditional methods that rely on spatial orientations, the proposed approach focuses on the proximity between data points by prioritizing the influence of regressor effects over spatial factors. The crux of this paper lies in the prescription and recommen- dations, which were derived using a customized algorithm, centered around the proximal data points. The proposed system exhibited notable accuracy rates when compared with three alternative approaches (kNN, Decision Tree, Naive Bayes) with accuracy rates of 97.4% and 89.6% in providing recommendations for response and input nutrients, respectively.
Keywords
Quantile Random Forests, Recommender systems, Prescriptive analytics, Proximity matrix
Cite as
Sareena Rose, S Nickolas and S Sangeetha, "Enhancing Precision Agriculture Through Novel Soil Nutrient Prescription Recommender System Using Quantile Regression Forests", In: Satyasai Jagannath Nanda and Rajendra Prasad Yadav (eds), Data Science and Intelligent Computing Techniques, SCRS, India, 2023, pp. 657-675. https://doi.org/10.56155/978-81-955020-2-8-59