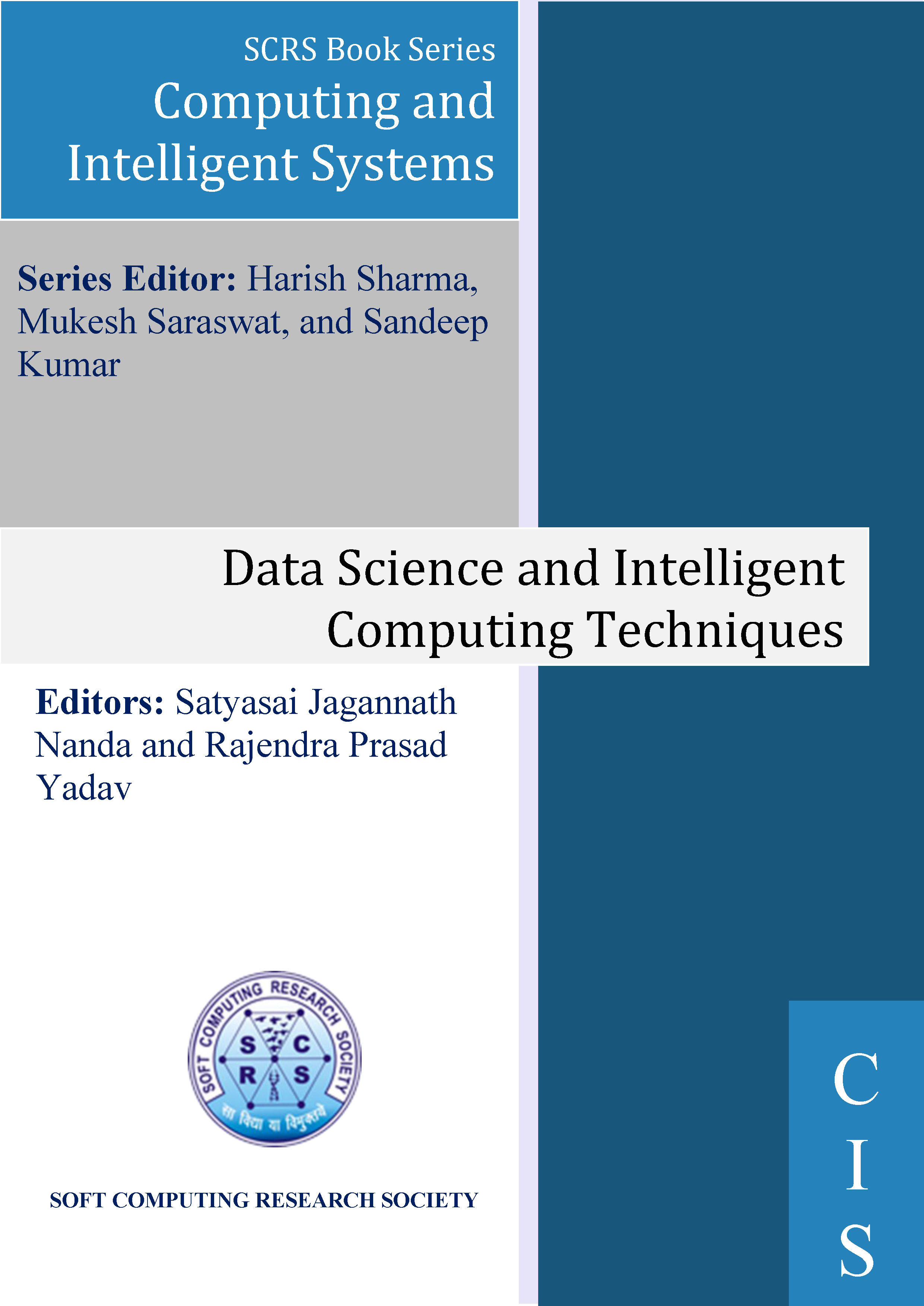
Causality in Time-Series: A Short Review
Authors: Girish Keshav Palshikar, Manoj Apte, Sushodhan Vaishampayan and Akshada Shinde
Publishing Date: 13-12-2023
ISBN: 978-81-955020-2-8
Abstract
The study of causal relations has been proved to be significant in acquisition, understanding, and representation of human knowledge across physical and biological sciences, engineering, social sciences, economics. Identifying or inferring causal relations from empirical data is a crucial step in knowledge acquisition. For study of causality, time is an important factor, as the effect will always occur after its cause, and, often, within stipulated amount of time. Thus, time-series data is considered ideal for causal inference. In this chapter we review various notions of causality in time-series data. Granger Causality is the most widely used notion for extraction of causal relations from time-series data, and has been extended to non-linear, conditional and multivariate scenarios. We also review some of the other notions of causality like Dynamic Bayesian network and Mutual Information related causal extraction techniques. We briefly touch upon Sir Austin Bradford Hill’s criteria for causality, which puts forward nine viewpoints for concluding an association relation into causality. Some applications of causality and a list of software tools are also provided. The chapter will provide sufficient information for the readers looking for a quick introduction to the field.
Keywords
Causality, Time-series data
Cite as
Girish Keshav Palshikar, Manoj Apte, Sushodhan Vaishampayan and Akshada Shinde, "Causality in Time-Series: A Short Review", In: Satyasai Jagannath Nanda and Rajendra Prasad Yadav (eds), Data Science and Intelligent Computing Techniques, SCRS, India, 2023, pp. 723-748. https://doi.org/10.56155/978-81-955020-2-8-64