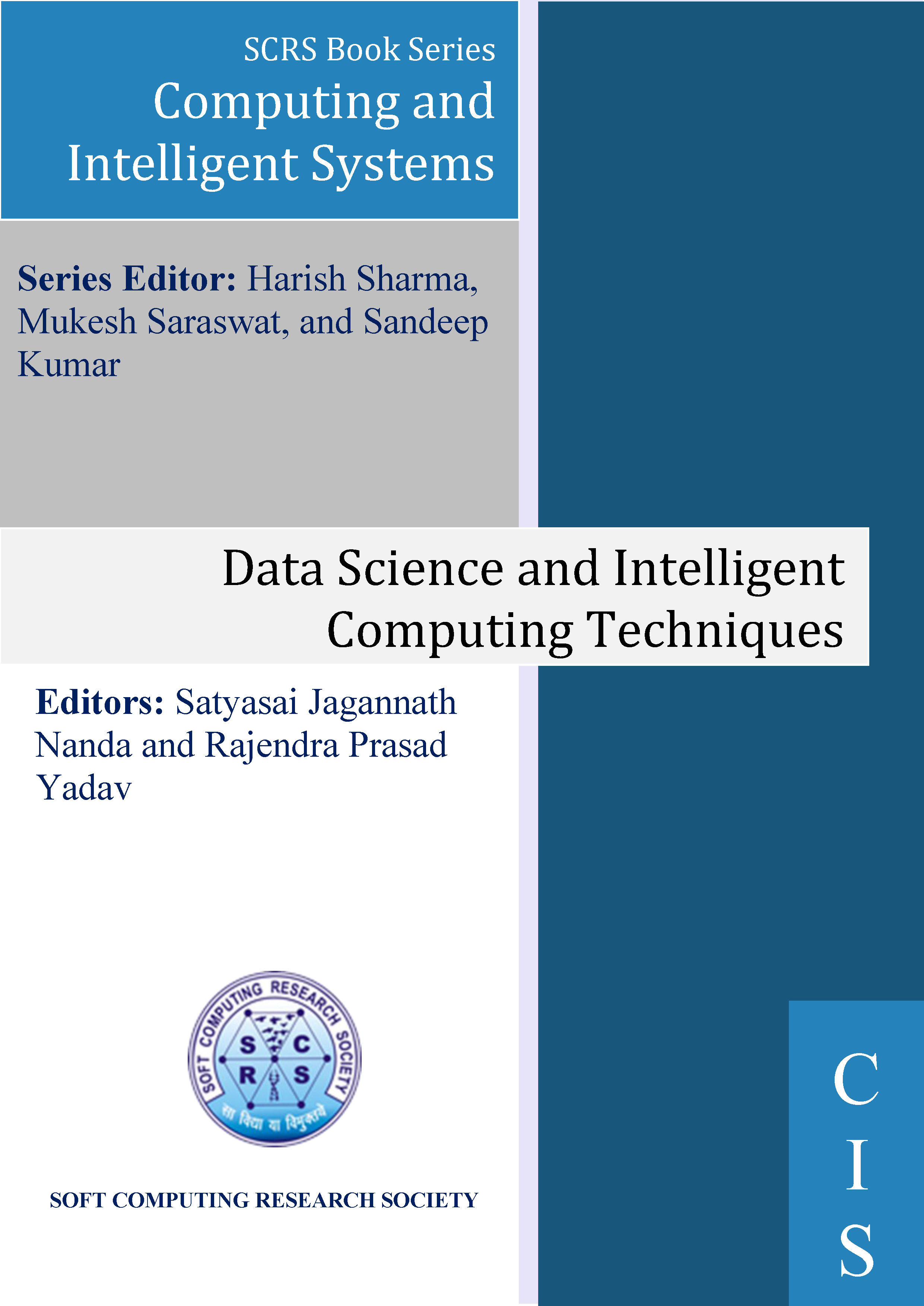
Managing Cold-start in the Serverless Cloud
Authors: Gunn Soni, Prince Kumar Singh, Mrinank Chandna and Shallu Rani
Publishing Date: 19-12-2023
ISBN: 978-81-955020-2-8
Abstract
Managing cold-start challenges in the serverless cloud environment is crucial for ensuring optimal performance and resource efficiency. This paper presents a comprehensive approach to address these challenges by integrating Temporal Convolutional Networks (TCNs) and Ensemble Policies, aiming to revolutionize the management of serverless cloud environments. The proposed framework leverages predictive models to anticipate infrastructure demands and function instance arrivals, enabling proactive resource provisioning and code optimization. A critical analysis, literature review, and methodological evaluation highlight the robustness and adaptability of the integrated approach. The ensemble policy’s parallel paths provide a versatile and scalable mechanism for addressing both infrastructure-level and function-level cold-start issues, resulting in improved resource allocation and minimized delays. This research significantly contributes to the advancement of cloud infrastructure management, offering valuable insights into optimizing serverless computing performance under varying workload conditions. Furthermore, the implementation analysis emphasizes the practical applicability of the proposed approach, demonstrating its potential to enhance overall system efficiency and responsiveness in dynamic and resource-constrained cloud environments.
Keywords
Cloud performance, cold-start, temporal convolutional networks, resource allocation, code optimization
Cite as
Gunn Soni, Prince Kumar Singh, Mrinank Chandna and Shallu Rani, "Managing Cold-start in the Serverless Cloud", In: Satyasai Jagannath Nanda and Rajendra Prasad Yadav (eds), Data Science and Intelligent Computing Techniques, SCRS, India, 2023, pp. 809-825. https://doi.org/10.56155/978-81-955020-2-8-69