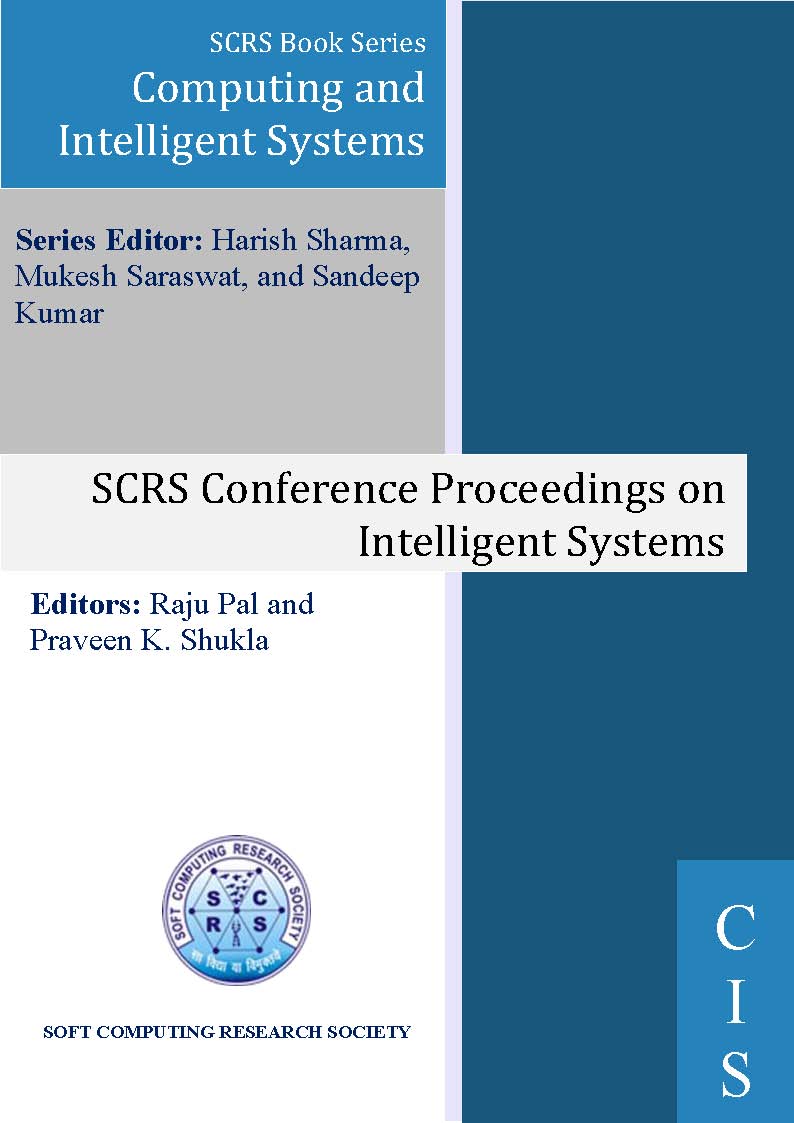
A Comprehensive survey on Various Machine Learning Models for Anomaly-Based Intrusion Detection System
Authors: Hidangmayum Satyajeet Sharma and Khundrakpam Johnson Singh
Publishing Date: 16-09-2022
ISBN: 978-81-955020-5-9
Abstract
As a result of the current global pandemic, there has been a surge in the use of various online platforms and services available via the internet. This results in the increase of exposure to different cyber-attacks on the network infrastructures. Cyber threats in the form of malicious software or also known as “malwares” have posed a serious threat to the smooth running of both government and business sectors. To cope with such issues, researchers have come up with various methods of machine learning based techniques in order to detect malicious activity on the network. But the major issue remains with the presence of vast diversity of features that leads to lengthy training processes and the need to deal with the prediction accuracy. This paper presents a literary review of various works on different machine learning-based intrusion detection system presented in different research papers over the last five years. Also, the results obtained from the various works such as evaluated metrics, datasets, and accuracy are discussed and compared. The scope of our review study is to provide a brief idea of intrusion detection as well as a reference to other research works done in the field of machine learning based intrusion detection system. Finally, the issues and future development are discussed by evaluating typical studies from recent years.
Keywords
Malware, Intrusion Detection System, DDoS attack, Machine Learning, Feature selection, Ensemble, Hybrid.
Cite as
Hidangmayum Satyajeet Sharma and Khundrakpam Johnson Singh, "A Comprehensive survey on Various Machine Learning Models for Anomaly-Based Intrusion Detection System", In: Saroj Hiranwal and Garima Mathur (eds), Artificial Intelligence and Communication Technologies, SCRS, India, 2022, pp. 393-408. https://doi.org/10.52458/978-81-955020-5-9-38